Cascaded Context-Aware Instance Segmentation with Transformer-Encoder for Adverse Weather Condition
Segmentasi Instansi Berbasis Konteks Bertingkat dengan Transformer-Encoder untuk Kondisi Cuaca Buruk
Abstract
Localizing objects from an image has been a vital part in autonomous driving since object localization performance directly correlate with the safety of the passenger. Robust and accurate object localization that can adapt to any driving environment has always been improved to ensure a safe and reliable system. In this work, we propose CBNet, a two-stage instance segmentation network for an autonomous driving environment. The network leverages a powerful transformer network as the feature extractor to improve performance. In addition, our proposed network utilizes a cascade design for both the object proposal network and the region-of-interests classifier. The cascade design addresses the issue of degrading detections over a high detection threshold. Moreover, we implement shape and edge-aware losses for the segmentation mask and end-to-end knowledge distillation strategy during training to improve the robustness of the network in extreme conditions. Our proposed network achieves 6.5 AP and 5.7 mIoU improvement from the prior methods in Cityscapes driving dataset. Furthermore, we evaluate our network in Foggy Cityscapes dataset to ensure the robustness of our network in extreme conditions. CBNet is able to improve the performance of prior methods by 7.7 AP and 6.7 mIoU in Foggy Cityscapes dataset.
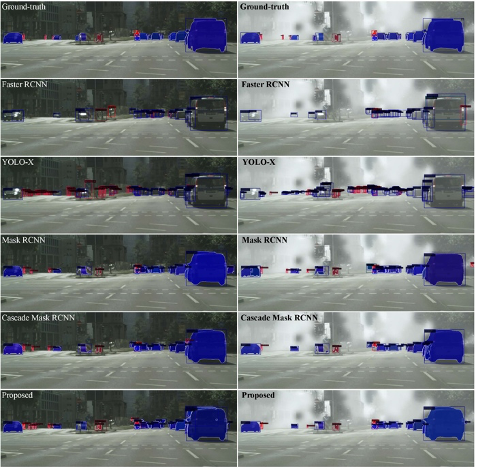
Copyright (c) 2024 Muhamad Amirul Haq, Le Nam Quoc Huy

This work is licensed under a Creative Commons Attribution 4.0 International License.