Classification of Student Complaints with the Naive Bayes and Literature Methods
Klasifikasi Keluhan Mahasiswa dengan Metode Naive Bayes dan Sastrawi
Abstract
E-Complaint Data is a collection of data which contains comments or complaints from students towards the University. Lots of comments - comments that occur in the university environment about the performance facilities of lecturers etc ... Text mining is also known as text data mining or knowledge search in a textual database is a semi-automatic process of extracting data patterns. The purpose of text mining is to get useful information from a collection of documents. In this study using the naïve Bayes method with TFIDF weighting features. The stages will be taken to determine its classification. First is taking data from the E-Complaint System then the data will go through the preprocessing stage using literary libraries, after going through the preprocessing stage the data will be divided into 2 namely training data and testing data. Then the training data will be carried out the TF-IDF weighting process up to the probability, if it has, the next is to process the testing data by determining priors. Next is the data testing stage between the testing data and the training data, the results of the testing data will come in the form of a predetermined category. The trial results show that the classification of complaints with the naïve Bayes algorithm and with the TF-IDF feature and literary libraries in the preprocessing process has an average accuracy that is quite high at 82%.
References
A. S. Fitriani, T. Informatika, F. Teknik, and U. M. Sidoarjo, “Penerapan Data Mining Menggunakan Metode Klasifikasi Naïve Bayes untuk Memprediksi Partisipasi Pemilihan Gubernur,” vol. 3, no. 2, pp. 98–104, 2019.
M. A. Rosid, G. Gunawan, and E. Pramana, “Centroid Based Classifier With TF – IDF – ICF for Classfication of Student’s Complaint at Appliation E-Complaint in Muhammadiyah University of Sidoarjo,” vol. 1, no. 1, 2015. DOI: https://doi.org/10.21070/jeee-u.v1i1.23
R. T. Wahyuni, D. Prastiyanto, and E. Supraptono, “Penerapan Algoritma Cosine Similarity dan Pembobotan TF-IDF pada Sistem Klasifikasi Dokumen Skripsi,” J. Tek. Elektro, vol. 9, no. 1, pp. 18–23, 2017.
A. Rachmat C and Y. Lukito, “Klasifikasi Sentimen Komentar Politik dari Facebook Page Menggunakan Naive Bayes,” J. Inform. dan Sist. Inf. Univ. Ciputra, vol. 2, no. 2, pp. 26–34, 2016, doi: 10.1080/10408398.2013.809690. DOI: https://doi.org/10.1080/10408398.2013.809690
S. Gusriani, K. D. K. Wardhani, and M. I. Zul, “Analisis Sentimen Terhadap Toko Online di Sosial Media Menggunakan Metode Klasifikasi Naïve Bayes (Studi Kasus: Facebook Page BerryBenka),” 4th Appl. Bus. Eng. Conf., vol. 1, no. 1, pp. 1–7, 2016.
Spr. Rani, B. Ramesh, and M. Anusha, “Evaluation of stemming techniques for text classification,” J. Comput. …, vol. 43, no. 3, pp. 165–171, 2015.
J. T. Informasi et al., “PENGARUH TEXT PREPROCESSING DAN KOMBINASINYA,” vol. 15, pp. 1–11, 2019.
S. Vijayarani, M. J. Ilamathi, M. Nithya, A. Professor, and M. P. Research Scholar, “Preprocessing Techniques for Text Mining -An Overview,” vol. 5, no. 1, pp. 7–16.
W. E. Nurjanah, R. S. Perdana, and M. A. Fauzi, “Analisis Sentimen Terhadap Tayangan Televisi Berdasarkan Opini Masyarakat pada Media Sosial Twitter menggunakan Metode K-Nearest Neighbor dan Analisis Sentimen Terhadap Tayangan Televisi Berdasarkan Opini Masyarakat pada Media Sosial Twitter menggunakan M,” no. October, 2017.
S. S. Nikam, “A Comparative Study of Classification Techniques in Data Mining Algorithms,” Int. J. Mod. Trends Eng. Res., vol. 4, no. 7, pp. 58–63, 2017, doi: 10.21884/ijmter.2017.4211.vxayk. DOI: https://doi.org/10.21884/IJMTER.2017.4211.VXAYK
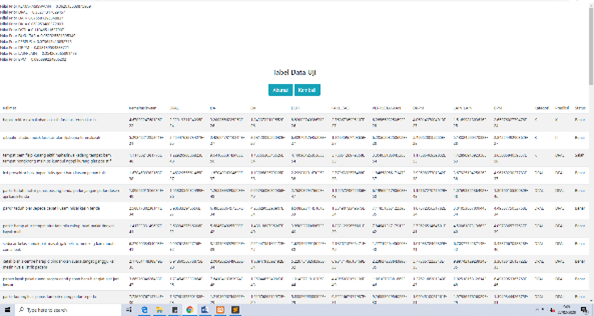
Copyright (c) 2020 Haris Ahmad Gozali, Mochamad Alfan Rosid, Sumarno

This work is licensed under a Creative Commons Attribution 4.0 International License.